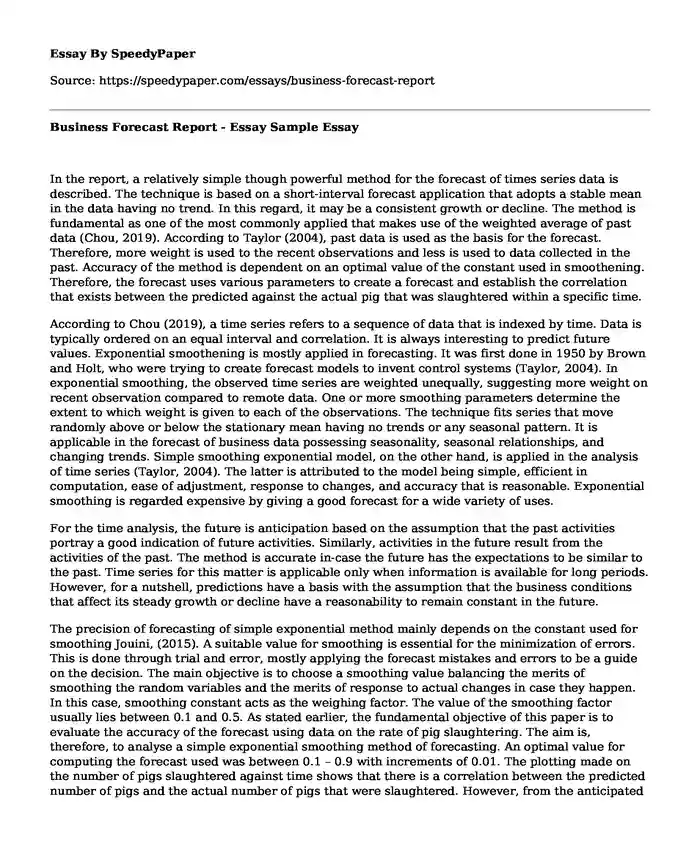
Type of paper:Â | Essay |
Categories:Â | Business |
Pages: | 4 |
Wordcount: | 1002 words |
In the report, a relatively simple though powerful method for the forecast of times series data is described. The technique is based on a short-interval forecast application that adopts a stable mean in the data having no trend. In this regard, it may be a consistent growth or decline. The method is fundamental as one of the most commonly applied that makes use of the weighted average of past data (Chou, 2019). According to Taylor (2004), past data is used as the basis for the forecast. Therefore, more weight is used to the recent observations and less is used to data collected in the past. Accuracy of the method is dependent on an optimal value of the constant used in smoothening. Therefore, the forecast uses various parameters to create a forecast and establish the correlation that exists between the predicted against the actual pig that was slaughtered within a specific time.
According to Chou (2019), a time series refers to a sequence of data that is indexed by time. Data is typically ordered on an equal interval and correlation. It is always interesting to predict future values. Exponential smoothening is mostly applied in forecasting. It was first done in 1950 by Brown and Holt, who were trying to create forecast models to invent control systems (Taylor, 2004). In exponential smoothing, the observed time series are weighted unequally, suggesting more weight on recent observation compared to remote data. One or more smoothing parameters determine the extent to which weight is given to each of the observations. The technique fits series that move randomly above or below the stationary mean having no trends or any seasonal pattern. It is applicable in the forecast of business data possessing seasonality, seasonal relationships, and changing trends. Simple smoothing exponential model, on the other hand, is applied in the analysis of time series (Taylor, 2004). The latter is attributed to the model being simple, efficient in computation, ease of adjustment, response to changes, and accuracy that is reasonable. Exponential smoothing is regarded expensive by giving a good forecast for a wide variety of uses.
For the time analysis, the future is anticipation based on the assumption that the past activities portray a good indication of future activities. Similarly, activities in the future result from the activities of the past. The method is accurate in-case the future has the expectations to be similar to the past. Time series for this matter is applicable only when information is available for long periods. However, for a nutshell, predictions have a basis with the assumption that the business conditions that affect its steady growth or decline have a reasonability to remain constant in the future.
The precision of forecasting of simple exponential method mainly depends on the constant used for smoothing Jouini, (2015). A suitable value for smoothing is essential for the minimization of errors. This is done through trial and error, mostly applying the forecast mistakes and errors to be a guide on the decision. The main objective is to choose a smoothing value balancing the merits of smoothing the random variables and the merits of response to actual changes in case they happen. In this case, smoothing constant acts as the weighing factor. The value of the smoothing factor usually lies between 0.1 and 0.5. As stated earlier, the fundamental objective of this paper is to evaluate the accuracy of the forecast using data on the rate of pig slaughtering. The aim is, therefore, to analyse a simple exponential smoothing method of forecasting. An optimal value for computing the forecast used was between 0.1 – 0.9 with increments of 0.01. The plotting made on the number of pigs slaughtered against time shows that there is a correlation between the predicted number of pigs and the actual number of pigs that were slaughtered. However, from the anticipated projection, the graph indicates that there was a variation in the number of pigs that were forecasted and the actual pigs that were slaughtered.
According to Chou (2019), the simple exponential smoothing technique gives an idea and a conclusion that the recent observations provide suitable guidance for the future. This calls for a weighting scheme having declining weights for the older data and observations. The decision on the smoothing constant to use is essential in the determination of the operational characteristics possessed by the exponential smoothing. A smaller value of the smoothing constant is an indication of a slow response. According to Chou (2019), a large value of the smoothing value is an indication of a quick reaction to actual changes as well as random changes.
As suggested by Jouini (2015), a simple exponential smoothing model is essential for non-seasonal patterns that have approximately zero trends as well as short term forecast. The reason is that an extension beyond the next period will cause the value for the forecast to be applied as a surrogate for the real demand for any prediction beyond the next period (Chou, 2019). On the same note, there exists no ability and possibility to add corrective information of any actual demand, and the error tends to grow exponentially. The future is taken to be an extension of the previous events. For a time, series under consideration, data available should be of more extended periods. In the case of the past trend being stable and steady, the future will be accurately predicted.
In conclusion, prediction and forecasts that are produced with the use of simple exponential smoothing techniques are weighted means of previous past observations. The weights decay exponentially as these observations get older. A clear indication is that recent observations have a higher weight. Prediction is the weighted total of previous observations. The SES model applies a decreasing weight for the past data.
References
Chou, M. (2019). Fuzzy forecast based on fuzzy time series. Time Series Analysis - Data, Methods, and Applications. https://doi.org/10.5772/intechopen.82843
Jouini, T. (2015). Efficient Multistep forecast procedures for multivariate time series. Journal of Forecasting, 34(7), 604-618. https://doi.org/10.1002/for.2363
Taylor, J. W. (2004). Smooth transition exponential smoothing. Journal of Forecasting, 23(6), 385-404. https://doi.org/10.1002/for.918
Cite this page
Business Forecast Report - Essay Sample. (2023, Dec 13). Retrieved from https://speedypaper.net/essays/business-forecast-report
Request Removal
If you are the original author of this essay and no longer wish to have it published on the SpeedyPaper website, please click below to request its removal:
- Exporting and Global Sourcing, Free Essay for Your Inspiration
- Event Evaluation Essay Sample
- Managing Customer Experience and Relationships. Essay Example
- Free Essay on Decision-Making and Consumer Behavior
- Essay Sample on Faith-Based Marketing
- Insurable Risks. Free Essay Example
- Essay Sample: The Argument for Limitation of Speed Limits
Popular categories