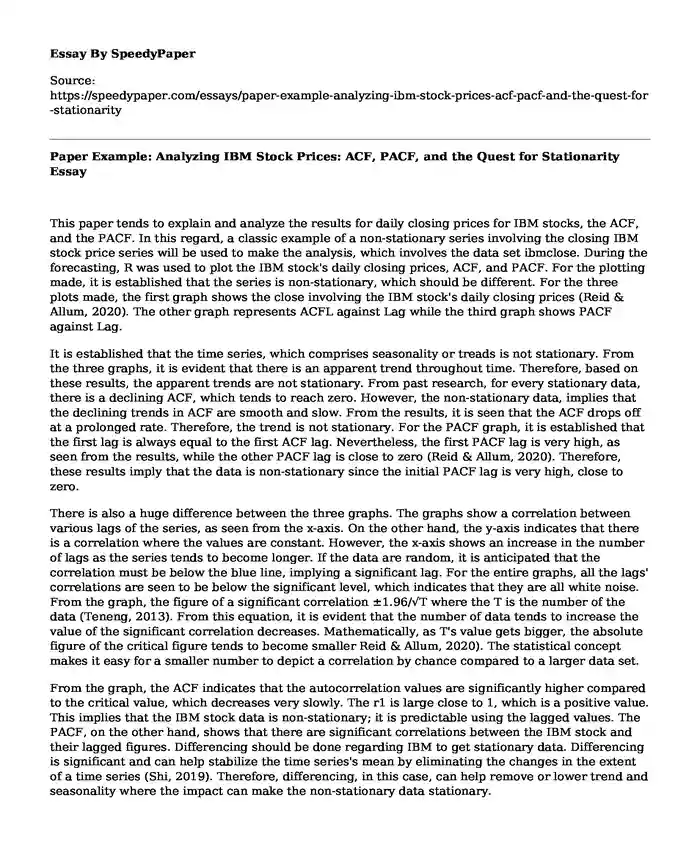
Type of paper: | Essay |
Categories: | Data analysis Statistics |
Pages: | 3 |
Wordcount: | 670 words |
This paper tends to explain and analyze the results for daily closing prices for IBM stocks, the ACF, and the PACF. In this regard, a classic example of a non-stationary series involving the closing IBM stock price series will be used to make the analysis, which involves the data set ibmclose. During the forecasting, R was used to plot the IBM stock's daily closing prices, ACF, and PACF. For the plotting made, it is established that the series is non-stationary, which should be different. For the three plots made, the first graph shows the close involving the IBM stock's daily closing prices (Reid & Allum, 2020). The other graph represents ACFL against Lag while the third graph shows PACF against Lag.
It is established that the time series, which comprises seasonality or treads is not stationary. From the three graphs, it is evident that there is an apparent trend throughout time. Therefore, based on these results, the apparent trends are not stationary. From past research, for every stationary data, there is a declining ACF, which tends to reach zero. However, the non-stationary data, implies that the declining trends in ACF are smooth and slow. From the results, it is seen that the ACF drops off at a prolonged rate. Therefore, the trend is not stationary. For the PACF graph, it is established that the first lag is always equal to the first ACF lag. Nevertheless, the first PACF lag is very high, as seen from the results, while the other PACF lag is close to zero (Reid & Allum, 2020). Therefore, these results imply that the data is non-stationary since the initial PACF lag is very high, close to zero.
There is also a huge difference between the three graphs. The graphs show a correlation between various lags of the series, as seen from the x-axis. On the other hand, the y-axis indicates that there is a correlation where the values are constant. However, the x-axis shows an increase in the number of lags as the series tends to become longer. If the data are random, it is anticipated that the correlation must be below the blue line, implying a significant lag. For the entire graphs, all the lags' correlations are seen to be below the significant level, which indicates that they are all white noise. From the graph, the figure of a significant correlation ±1.96/√T where the T is the number of the data (Teneng, 2013). From this equation, it is evident that the number of data tends to increase the value of the significant correlation decreases. Mathematically, as T's value gets bigger, the absolute figure of the critical figure tends to become smaller Reid & Allum, 2020). The statistical concept makes it easy for a smaller number to depict a correlation by chance compared to a larger data set.
From the graph, the ACF indicates that the autocorrelation values are significantly higher compared to the critical value, which decreases very slowly. The r1 is large close to 1, which is a positive value. This implies that the IBM stock data is non-stationary; it is predictable using the lagged values. The PACF, on the other hand, shows that there are significant correlations between the IBM stock and their lagged figures. Differencing should be done regarding IBM to get stationary data. Differencing is significant and can help stabilize the time series's mean by eliminating the changes in the extent of a time series (Shi, 2019). Therefore, differencing, in this case, can help remove or lower trend and seasonality where the impact can make the non-stationary data stationary.
References
Reid, A., & Allum, N. (2020). Learn about time series ACF and PACF in Stata with data from the NOAA global climate at a glance (1910–2015). https://doi.org/10.4135/9781529710397
Shi, F. (2019). Learn about time series ACF and PACF in SPSS with data from EPA’s air quality system data mart (2017). https://doi.org/10.4135/9781526468697
Teneng, D. (2013). Modeling and forecasting foreign exchange daily closing prices with normal inverse gaussian. https://doi.org/10.1063/1.4823953
Cite this page
Paper Example: Analyzing IBM Stock Prices: ACF, PACF, and the Quest for Stationarity. (2023, Dec 20). Retrieved from https://speedypaper.net/essays/paper-example-analyzing-ibm-stock-prices-acf-pacf-and-the-quest-for-stationarity
Request Removal
If you are the original author of this essay and no longer wish to have it published on the SpeedyPaper website, please click below to request its removal:
- Free Botanists Essay Example
- Essay Sample on How Good Architectural Designs Impact People's Lives
- Dembski's Book: Religion, Science & Intelligent Design. Essay Example
- Paper Example. A Priori Qualitative Research Article
- Essay Sample on The Advantages and Disadvantages of GAP 43
- US-China Relations: Reflections on a Gathering Storm - Essay Sample
- Argumentative Essay: Social Media and the False Claims About Trump's Administration
Popular categories