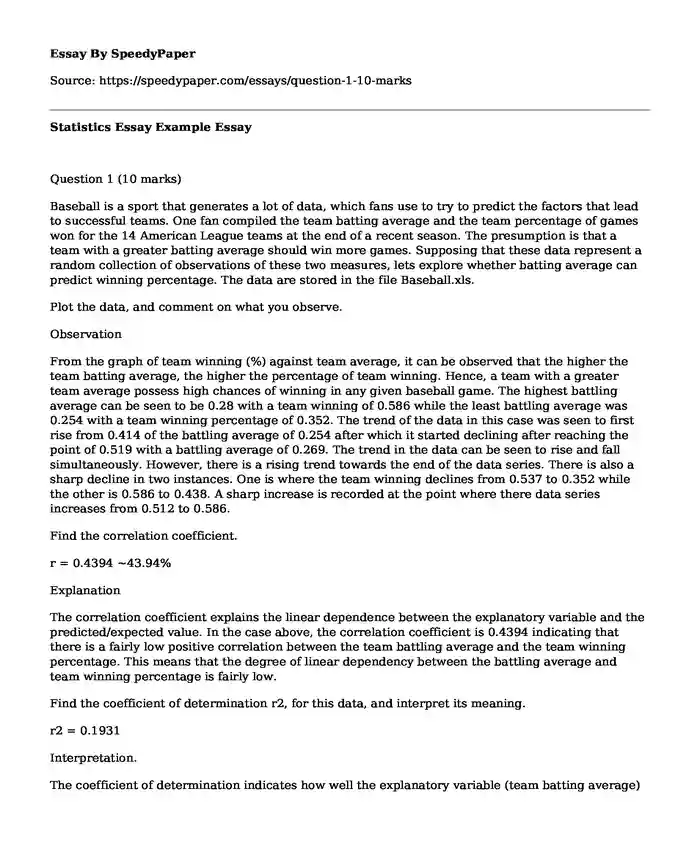
Type of paper:Â | Essay |
Categories:Â | Data analysis Statistics |
Pages: | 7 |
Wordcount: | 1675 words |
Question 1 (10 marks)
Baseball is a sport that generates a lot of data, which fans use to try to predict the factors that lead to successful teams. One fan compiled the team batting average and the team percentage of games won for the 14 American League teams at the end of a recent season. The presumption is that a team with a greater batting average should win more games. Supposing that these data represent a random collection of observations of these two measures, lets explore whether batting average can predict winning percentage. The data are stored in the file Baseball.xls.
Plot the data, and comment on what you observe.
Observation
From the graph of team winning (%) against team average, it can be observed that the higher the team batting average, the higher the percentage of team winning. Hence, a team with a greater team average possess high chances of winning in any given baseball game. The highest battling average can be seen to be 0.28 with a team winning of 0.586 while the least battling average was 0.254 with a team winning percentage of 0.352. The trend of the data in this case was seen to first rise from 0.414 of the battling average of 0.254 after which it started declining after reaching the point of 0.519 with a battling average of 0.269. The trend in the data can be seen to rise and fall simultaneously. However, there is a rising trend towards the end of the data series. There is also a sharp decline in two instances. One is where the team winning declines from 0.537 to 0.352 while the other is 0.586 to 0.438. A sharp increase is recorded at the point where there data series increases from 0.512 to 0.586.
Find the correlation coefficient.
r = 0.4394 ~43.94%
Explanation
The correlation coefficient explains the linear dependence between the explanatory variable and the predicted/expected value. In the case above, the correlation coefficient is 0.4394 indicating that there is a fairly low positive correlation between the team battling average and the team winning percentage. This means that the degree of linear dependency between the battling average and team winning percentage is fairly low.
Find the coefficient of determination r2, for this data, and interpret its meaning.
r2 = 0.1931
Interpretation.
The coefficient of determination indicates how well the explanatory variable (team batting average) explains the dependent variable (percentage of team winning)
In the case above:
The r2 (coefficient of determination) represents the goodness of fit. It implies team batting average accounts for 19.31% of all the changes in the percentage of team winning holding other factors constant. Hence, there is about 80.69% of the changes in the percentage of team winning that is explained by other factors and the error term.
Find the sample regression line, and interpret the meaning of the coefficients of your equation.
Sample Regression line: Y = a+bx
Y = -0.2268 + 2.7941X
Team winning (%) = -0.2268 + 2.7941Team battling average
Interpretation
a = -0.2268
Holding the team batting average constant (x=0), the expected value or the mean % of team winning is -0.2268.
b = 2.7941 (Y/X) = 2.7941
An increase in the team batting average by one additional point leads to an increase in the expected value of team winning by 2.7941 holding other factors constant.
Is there evidence at a 5% level of significance, that batting average can be used to predict winning percentage?
Ho: b = 0
HA: b 0
Significance level of b = b (ta/2 * Seb)
Where;
b = 2.7941
n = 14
k = 2
Degrees of freedom = n-k
d.f = 14-2
d.f = 12
Seb = 1.6490
For a two tailed test
a/2 = 5%/2
a = 2.5% ~0.025
Critical t-statistic = t0.025, 12d.f = 2.179
Tcalculated Computation
t = b b*
Seb
t = 2.7941 - 0
1.6490
tcalc = 1.6944
Decision criteria
Tcalc < Tcritical :Refuse to reject Ho; b- not statistically significant
1.6944 < 2.179
Conclusion
Since the calculated t is less than the critical t (Tcalc < Tcritical), we refuse to reject that Ho that b is not statistically significant and accept that b is not statistically significant and hence conclude that the batting average cannot be used to predict winning percentage.
The slope coefficient is equal to zero and hence it is not statistically significant thus the conclusion that the team batting average does not influence the team winning (%) and cannot be used in predicting the team winning (%)
Question 2 (10 marks)
Physicians are recommending more exercise for patients, especially those who are overweight. One benefit of regular exercise is thought to be a reduction of bad cholesterol. To study the relationship, a doctor selected a sample of patients who did not do regular exercise, and measured their cholesterol level. She then started the patients on a program of exercise, and asked them to record the number of minutes per week that they exercised. After 4 months, she re-measured their cholesterol levels. The data are contained in the file Cholesterol.xls.
Plot the data. Does it appear that amount of exercise and cholesterol level change is related?
After plotting the data on cholesterol level (before and after) against the amount of exercise (min), it can be found out that a decrease in the amount of exercise (min) leads to an increase in the cholesterol level. Thus there is a negative relationship between the amount of exercise and the cholesterol level. Thus, a change in the amount of exercise leads to a change in the cholesterol level hence the conclusion that the amount of exercise and cholesterol level change is related.
Determine the regression equation relating cholesterol reduction to amount of exercise, and find a 95% confidence interval for the intercept. Provide a brief and meaningful written interpretation of the coefficients and the confidence interval.
Regression line: Y = a + bx
Before
Y = 239.772 + 0.019 X
Cholesterol level = 239.772 + 0.019 Amount of exercise
After
Y = 237.722 0.0717X
Cholesterol level = 237.722 0.0717 Amount of exercise
CONFIDENCE IINTERVAL for the intercept
Cholesterol level before exercise
Confidence interval of a = a (za/2 * Seb)
Where;
a = 239.772
n = 50
k = 2
Degrees of freedom = n-k
d.f = 50-2
d.f = 48
Seb = 10. 1303
For a two tailed test
a= 5%
C.I = 1- a = 95% ~ 0.95
Critical z-statistic
z = (1.64 + 1.65)/2
z= 3.29/2
z= 1.645
Computation
C.I = a (za/2*Sea) b b + (za/2*Se a)
239.772 (1.645*10. 1303) a 239.772 + (1.645*10. 1303)
239.772 16.6643 a 239.772 + 16.6643
223.1077 a 256.4363
171450203835
95%
223.1077 a 256.4363
Explanation
The estimated value of a is 239.772 and we can be 95% confident that this value could range from 223.1077 to 256.4363.
Cholesterol level after exercise
Confidence interval of a = a ta/2 * Seb)
Where;
a = 237.7224
n = 50
k = 2
Seb = 10. 77
For a two tailed test
a= 5%
C.I = 1- a = 95% ~ 0.95
Critical z-statistic
z = (1.64 + 1.65)/2
z= 3.29/2
z= 1.645
Computation
C.I = a (t a/2*Sea) a a + (t a/2*Sea)
237.7224 (1.645*10. 77) a 237.7224 + (1.645*10. 77)
237.7224 17.7167 a 237.7224 + 17.7167
220.0057 a 255.4891
171450203835
95%
220.0057 a255.4891
Explanation
The estimated value of a is 237.7224 but we are 95% confident that this value could range from 220.0057 to 255.4891.
Can we conclude that exercise affects the change in cholesterol level of the exerciser?
Hypothesis
Before
HO: a = 245.22 model is not statistically significant
HA: a 245.22 model is statistically significant
After
HO: a = 217.42 model is not statistically significant
HA: a 217.42 model is statistically significant
After
a = 237.7224
Since the hypothesized value of the intercept (a) falls outside the confidence interval, hence, we reject Ho
Conclusion
The intercept is not equal to 217.42 and hence it is statistically significant and thus the amount of exercises performed affects the cholesterol level.
How well does the linear model fit this data? Justify.
In order to determine how well the linear model fits the data, the goodness of fit measure (coefficient of determination: r2) will be used. The coefficient of determination tells us how well the explanatory variable (which in this case is exercise) explains the dependent variable (which is the cholesterol level)
Exercise Before
r2 = 0.00698
The results above imply that number of exercises performed explain about 0.698% of all the variations in cholesterol levels holding other factors constant. In the above case, r2 = 0.00698 indicating that the exercises performed per (min) explain 0.698% of all the changes in the cholesterol level holding other factors constant. Hence, there is about 99.993% of the changes in cholesterol level explained by other factors and the error term.
Exercise After
r2 = 0.0795
The results above imply that number of exercises performed explain about 7.95% of all the variations in cholesterol levels holding other factors constant. In the above case, r2 = 0.0795 indicating that the exercises performed per (min) explain 7.95% of all the changes in the cholesterol level holding other factors constant. Hence, there is about 92.05% of the changes in cholesterol level explained by other factors and the error term.
Question 3 (10 marks)
Hardwood trees are harvested in a selective manner for the manufacture of fine furniture. Environmental groups are concerned that as few trees are selected for cutting as possible while companies feel that they need a certain amount of wood for manufacturing. To help each group predict the volume of lumber in a selected tree, various measurements are made before the tree is cut. Unfortunately, volume is not easily determined before harvesting.
Two common measurements made before cutting down the tree are DBH (the diameter of the tree at breast height, 4.5 feet off the ground) and the height of the tree measured with sighting instruments. After the tree is harvested the volume of lumber may be measured.
Both groups believe that a regression model relating volume to diameter and/or height will be helpful. The data file below gives the diameters, heights, and volumes of 31 trees harvested in the Allegheny National Forest in Pennsylvania. The data are contained in the file Wood.xlsx.
Estimate the two simple regression models and the multiple regression model that is appropriate for these data.
1. Simple Regression model
Volume vs diameter
Y = -36.9435 + 5.0659 X
Volume = -36.9435 + 5.0659 Diameter
Volume vs height
Y = -87.1236 + 1.5433
Volume = -87.1236 + 1.5433 Height
2. Multiple regression model
Y = -57. 9877 + 4.7082 B1 +0.3393 B2
Volume = -57. 9877 + 4.7082 Diameter + 0.3393 Height
Which model would you recommend that the two groups use? Why?
Simple regression
Volume vs diameter
Multiple r = 0.9671
r2 = 0.9353
Volume vs height
r = 0.5982
r2 = 0.3579
Multiple regression
Multiple r = 0.9736
R2 = 0.94795
Adjusted r2 = 0.9442
The multiple regression model is more useful for use and hence it would be more favorable to utilize in forecasting. This is because multi regression has a higher correlation coefficient between the diameter and height. Additionally, multi regression provides better results in terms of the goodness of fit. The outcome obtained from multi regression equation provides for a higher coefficient of determination (r2 =0.94795) making it a better model to use in estimation. The diameter and the height jointly explain 94.8% of all the variations in the volume holding other factors constant. When using the adjusted (r2 = 94.42) the diameter and height jointly explain about 94.42% of all the variations in the volume adjusted by the degrees of freedom.
A tree with a height of 72 and a diameter of 15.9 has just arrived at the mill. What v...
Cite this page
Statistics Essay Example. (2019, May 15). Retrieved from https://speedypaper.net/essays/question-1-10-marks
Request Removal
If you are the original author of this essay and no longer wish to have it published on the SpeedyPaper website, please click below to request its removal:
- Meaning of Altruism - Essay Sample
- Essay Sample on Network Security
- Essay Sample on Mandated Curriculum for Training Children
- Free Essay Sample: Bioenergy and Its Environmental Properties
- Powers of the National Government, Free Essay for Everyone
- Drivers for Sustainable Construction Essay Sample
- Essay Example on Apple Inc. in a Competitive Environment
Popular categories