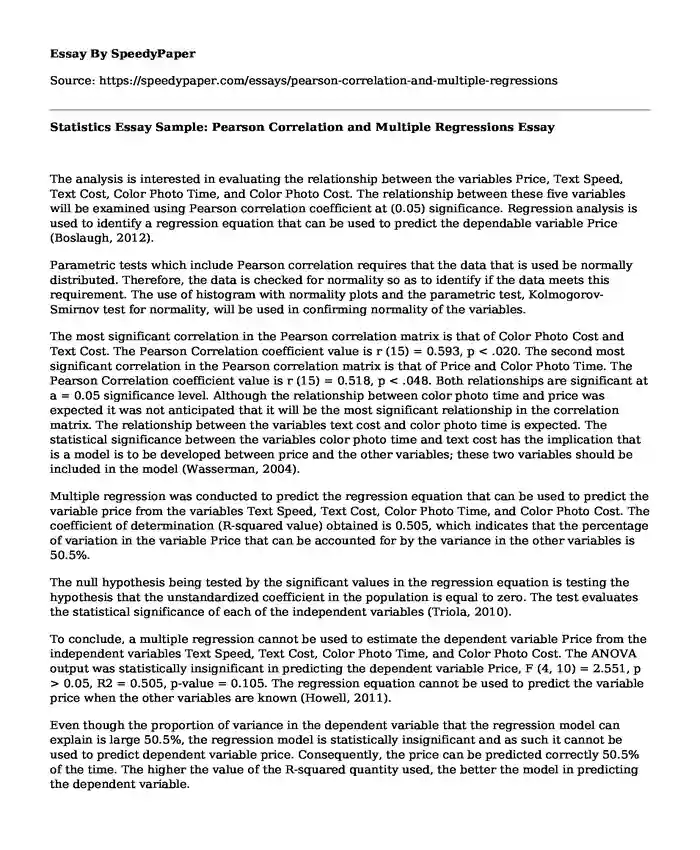
Type of paper:Â | Essay |
Categories:Â | Data analysis Statistics |
Pages: | 5 |
Wordcount: | 1141 words |
The analysis is interested in evaluating the relationship between the variables Price, Text Speed, Text Cost, Color Photo Time, and Color Photo Cost. The relationship between these five variables will be examined using Pearson correlation coefficient at (0.05) significance. Regression analysis is used to identify a regression equation that can be used to predict the dependable variable Price (Boslaugh, 2012).
Parametric tests which include Pearson correlation requires that the data that is used be normally distributed. Therefore, the data is checked for normality so as to identify if the data meets this requirement. The use of histogram with normality plots and the parametric test, Kolmogorov-Smirnov test for normality, will be used in confirming normality of the variables.
The most significant correlation in the Pearson correlation matrix is that of Color Photo Cost and Text Cost. The Pearson Correlation coefficient value is r (15) = 0.593, p < .020. The second most significant correlation in the Pearson correlation matrix is that of Price and Color Photo Time. The Pearson Correlation coefficient value is r (15) = 0.518, p < .048. Both relationships are significant at a = 0.05 significance level. Although the relationship between color photo time and price was expected it was not anticipated that it will be the most significant relationship in the correlation matrix. The relationship between the variables text cost and color photo time is expected. The statistical significance between the variables color photo time and text cost has the implication that is a model is to be developed between price and the other variables; these two variables should be included in the model (Wasserman, 2004).
Multiple regression was conducted to predict the regression equation that can be used to predict the variable price from the variables Text Speed, Text Cost, Color Photo Time, and Color Photo Cost. The coefficient of determination (R-squared value) obtained is 0.505, which indicates that the percentage of variation in the variable Price that can be accounted for by the variance in the other variables is 50.5%.
The null hypothesis being tested by the significant values in the regression equation is testing the hypothesis that the unstandardized coefficient in the population is equal to zero. The test evaluates the statistical significance of each of the independent variables (Triola, 2010).
To conclude, a multiple regression cannot be used to estimate the dependent variable Price from the independent variables Text Speed, Text Cost, Color Photo Time, and Color Photo Cost. The ANOVA output was statistically insignificant in predicting the dependent variable Price, F (4, 10) = 2.551, p > 0.05, R2 = 0.505, p-value = 0.105. The regression equation cannot be used to predict the variable price when the other variables are known (Howell, 2011).
Even though the proportion of variance in the dependent variable that the regression model can explain is large 50.5%, the regression model is statistically insignificant and as such it cannot be used to predict dependent variable price. Consequently, the price can be predicted correctly 50.5% of the time. The higher the value of the R-squared quantity used, the better the model in predicting the dependent variable.
In the sequence of model fitting, variables that were not significant in predicting price were removed from the regression equation through use of computed probabilities. The removal of variables with insignificant probabilities led to a model that was significant in predicting the price (Mardia, & Jupp, 1999).
Looking at the excluded model the variable that was almost included in the regression equation is the Text speed because it had the least significance as compared to the other excluded variables. Additionally, it was the last variable to be excluded based on the backward criterion (Field, 2013).
The regression model that is significant in predicting the price of the printer is
Price = 239.8 9.7 (Color Photo Time)
The final model cannot be used to predict the time it takes to reduce the text speed because the variable text speed is not included in the model that predicts the price. The absence of text speed as one of the independent variables makes the use of the regression equation to predict the cost of reducing text speed impossible. The use of a regression equation is only possible if the values of the independent variables used are known.
If the final model is used, the cost of reducing the time taken to print a color photograph by one second is 230.1 pounds
Price = 239.8 -9.7(1) =230.1 pounds.
The final model is computed using the backward criterion that uses the probability of the F statistic to eliminate insignificant independent variables. The model that consists of price as the dependent variable and color photo time as the independent variable is detailed below.
In conclusion, a multiple regression cannot be used to estimate the dependent variable Price from the independent variable Color Photo Time. The ANOVA output was statistically significant in predicting the dependent variable Price, F (1, 13) = 4.755, p > 0.05, R2 = 0.268, p-value = 0.048. The regression equation can be used to predict the variable price when the other variables are known because the probability value is less than the significance level used. The coefficient of determination value is small but, nonetheless, the regression equation is statistically significant in predicting the dependent variable.
References:
Boslaugh, S. (2012). Statistics in a nutshell. Sebastopol, CA: O'Reilly Media.
Field, A. (2013). Discovering Statistics using IBM SPSS Statistics.Howell, D. C. (2011). Fundamental statistics for the behavioral sciences. Belmont, CA: Wadsworth Cengage Learning.
Mardia, K. V., & Jupp, P. E. (1999). Directional Statistics. Chichester: John Wiley & Sons.
Triola, M. F. (2010). Elementary statistics. Boston: Addison-Wesley.
Wasserman, L. A. (2004). All of statistics: A concise course in statistical inference. New York, NY [u.a.: Springer.
Appendix 1
Table 1
Descriptive statistics
Information Mean Standard deviation N
Price 168.67 76.52 15 Text Speed 3.54 1.23 15 Text Cost 6.93 4.061 15 Color Photo Time 7.33 4.082 15 Color Photo Cost 1.1467 0.422 15 Table 2
Model summary
Model R R Square Adjusted R square Std. Error of the Estimate
1 0.711 0.505 0.307 63.696
Table 3
Correlations
Price Color Photo Time Text Cost Color Photo Cost
Price Pearson correlation Sig (2-tailed) 1 -0.518
0.048 -0.501
0.057 -0.263
0.158
Color Photo Time Pearson correlation Sig (2-tailed -0.263
0.158 1 0.398
0.142 0.081
0.773
Text Cost Pearson correlation Sig (2-tailed) -0.501
0.057 0.398
0.142 1
0.81
0.773
Color Photo Cost Pearson correlation Sig (2-tailed) -0.263
0.343 0.081
0.773 -0.713
0.000 1
Table 3
ANOVA
Model Sum of Squares dfMean Square F Sig
Regression 41402.112 4 10350.53 2.551 0.105
Residual 40571.221 10 4057.122 Total 81973.33 14 Table 4
Coefficients
Unstandardized Coefficients Standardized Coefficients Model B Std. Error Beta t Sig
Constant 334.167 68.425 4.884 0.001
Text Speed -23.475 14.344 -0.378 -1.637 0.133
Color Photo Cost 16.07 53.114 0.089 0.303 0.768
Color photo time -6.586 4.664 -0.351 -1.412 0.188
Text Cost -7.576 -5.852 -0.402 -1.295 0.225
Table 5
Model summary
Model R R Square Adjusted R square Std. Error of the Estimate
1 0.711 0.505 0.307 63.696
2 0.707 0.501 0.364 61.009
3 0.633 0.401 0.302 63.952
4 0.518 0.268 0.212 67.947
Table 6
ANOVA
Model Sum of Squares dfMean Square F Sig
1 Regression 41402.112 4 10350.53 2.551 0.105
Residual 40571.221 10 4057.122 Total 81973.33 14 2 Regression 41030.707 3 13676.902 3.675 0.047
Residual 40942.627 11 3722.057 Total 81973.33 14 3 Regression 32895.662 2 16447.81 4.022 0.046
Residual 49077.672 12 4089.806 Total 81973.33 14 4 Regression 21954.333 1 21954.333 4.755 0.048
Residual 60019 13 4616.846 Total 81973.333 14
Table 7
Coefficients
Unstandardized Coefficients Standardized Coefficients Model B Std. Error Beta t Sig
Constant 334.167 68.425 4.884 0.001
Text Speed -23.475 14.344 -0.378 -1.637 0.133
Color Photo Cost 16.07 53.114 0.089 0.303 0.768
Color photo time -6.586 4.664 -0.351 -1.412 0.188
Text Cost -7.576 -5.852 -0.402 -1.295 0.225
Constant 343.147 59.053 5.811 0.000
Text Speed -22.321 13.244 -0.359 -1.685 0.120
Color photo time -6.900 4.354 -0.368 -1.585 0.141
Text Cost -6.470 4.377 -0.343 -1.478 0.167
Constant 318.382 59.359 5.364 0.000
Text Speed -22.702 13.88 -0.366 -1.636 0.128
Color photo time -9.457 4.189 -0.505 -2.257 0.043
Constant 239.8 37.039 6.474 0.000
Color Photo Time -9.7 4.448 -0.518 -2.181 0.048
Cite this page
Statistics Essay Sample: Pearson Correlation and Multiple Regressions. (2019, Aug 30). Retrieved from https://speedypaper.net/essays/pearson-correlation-and-multiple-regressions
Request Removal
If you are the original author of this essay and no longer wish to have it published on the SpeedyPaper website, please click below to request its removal:
Popular categories