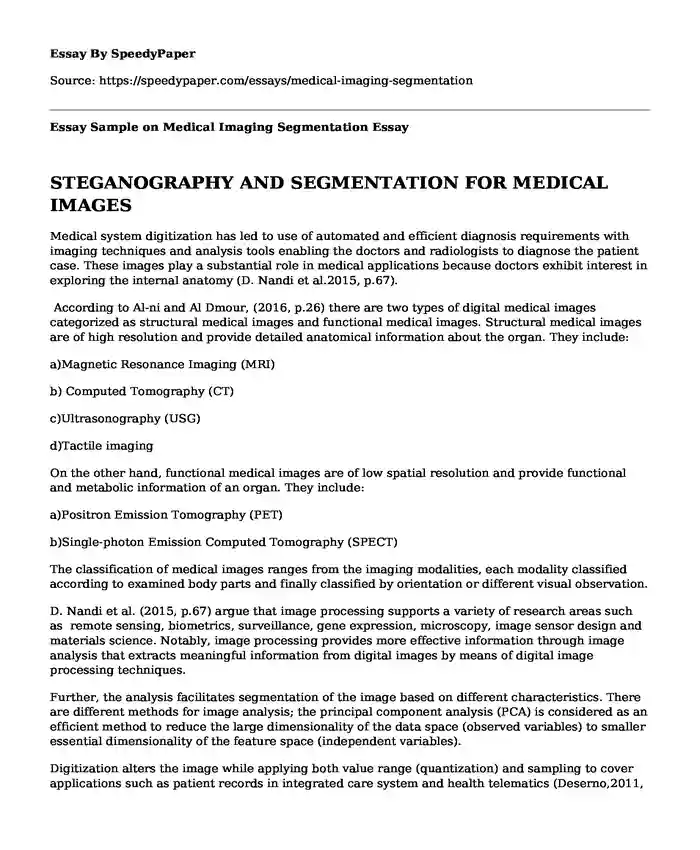
Type of paper:Â | Essay |
Categories:Â | Health and Social Care Medicine Technology |
Pages: | 6 |
Wordcount: | 1573 words |
STEGANOGRAPHY AND SEGMENTATION FOR MEDICAL IMAGES
Medical system digitization has led to use of automated and efficient diagnosis requirements with imaging techniques and analysis tools enabling the doctors and radiologists to diagnose the patient case. These images play a substantial role in medical applications because doctors exhibit interest in exploring the internal anatomy (D. Nandi et al.2015, p.67).
According to Al-ni and Al Dmour, (2016, p.26) there are two types of digital medical images categorized as structural medical images and functional medical images. Structural medical images are of high resolution and provide detailed anatomical information about the organ. They include:
a)Magnetic Resonance Imaging (MRI)
b) Computed Tomography (CT)
c)Ultrasonography (USG)
d)Tactile imaging
On the other hand, functional medical images are of low spatial resolution and provide functional and metabolic information of an organ. They include:
a)Positron Emission Tomography (PET)
b)Single-photon Emission Computed Tomography (SPECT)
The classification of medical images ranges from the imaging modalities, each modality classified according to examined body parts and finally classified by orientation or different visual observation.
D. Nandi et al. (2015, p.67) argue that image processing supports a variety of research areas such as remote sensing, biometrics, surveillance, gene expression, microscopy, image sensor design and materials science. Notably, image processing provides more effective information through image analysis that extracts meaningful information from digital images by means of digital image processing techniques.
Further, the analysis facilitates segmentation of the image based on different characteristics. There are different methods for image analysis; the principal component analysis (PCA) is considered as an efficient method to reduce the large dimensionality of the data space (observed variables) to smaller essential dimensionality of the feature space (independent variables).
Digitization alters the image while applying both value range (quantization) and sampling to cover applications such as patient records in integrated care system and health telematics (Deserno,2011, p.1).The digitization of medical system has allowed for the development of some of the analog imaging modalities such as radiography and endoscopy with set digital sensors. In analyzing the components of digital images, (Deserno, 2011, p.2) states that critical composition is individual pixels (element and picture) allocated separate color values. Subject to Picture Archiving and Communication System (PACS) and the digital imaging and communications in medicine protocols and communication, digital image processing is applicable is medicine. As a result, the pixels can be processed, assessed and made available to many places at the same time.
The four main areas covered by digital imaging to which the algorithms of image enhancement allocated as pre-processing and post-processing consist of image formation which entails capturing the image to forming a digital matrix. Secondly is analysis and management that is concerned with all processing processes used for quantitative measurements not to mention abstract interpretations of biomedical images. Thirdly, the image visualization that results to the optimized output of the picture. Lastly is the image management that provides efficient communication, transmission, storage and archiving as well as retrieval of image data in telemedicine (Deserno, 2011, p.3).
MRI is purely composed of electromagnetic effects of the nucleus. An example of MRI application would be to regard probation of tissue consisting of billions of hydrogen atoms. Through the microscopic view, the spins amount to microscopic magnetic moment M. The CT digital machine uses X-Ray to determine the absorption of images in 3D for each volume element (voxel). It acquires a volume slice by slice, reconstructs it in proper angulation. An example application would be where a slice divided into two parallel rays passing it in two different directions or four pixels; the results would be in four independent equations to allow four absorption coefficients
The importance of medical image processing
According to Al-ni and Al Dmour (2016, p. 24), currently, the health care system exploits the use of the internet to aid in the exchange of information, diagnosis and digital images between hospitals as they provide services to patients. Through processing, the hospitals can ensure secure storage and analysis as they seek to diagnose the right disease and treat the same. Some of the techniques used include MRI, tactile imagery, CT, SPECT and PET. Further, it provides innovation for medical imaging in modern health care, especially in bioinformatics, neuroinformatics, and informatics. Image processing allows for medical imaging in therapeutic applications and instrumentation. As argued by (Deserno and Handels et al.2013, p.3) image processing has increased acquisition of medical images using novel imaging modalities. Also, it aids in enhancement, a good example being from nano to macro as advocated by The Institute of Electrical and Electronics Engineers (IEEE) as it covers all aspects of medical imaging. Moreover, image processing is important in segmentation, registration of section images by use of auto radiology or histology imaging techniques based on thin post-mortem sections. It is also important in visualization and quantification. Lastly, used in computer-aided detection (CAD). Moreover, (D. Nandi et al., 2015, p.66) opines that image processing contributes to research areas such as image sensor design, material science, biometrics, gene expression, microscopy among others. Further, they enable radiologist and doctors to diagnose the patient case as mentioned.
The steganography (security)
As stated by (Mohapatra and Pandey, 2015, p.1), steganography entails secret communication. In the digital medical era where sharing of information has been made accessible, steganography keeps the message in secret. The information is hidden in the multimedia carrier like the image, video, and audio. The message is encrypted, only retrieved by use of pixel value that follows a particular procedure. One of the disadvantages of a digital system is the hacking and exposure of private information such as patient's disease and medical records. The security embeds patient’s information, details, and images (Mohapatra and Pandey. 2015, p.3).
Moreover, (Mohapatra and Pandey, 2015, p.3) provide that steganography is used to connect a website and download JPEG or HTML files earlier encoded under the private key. It allows for HTML or JPEG stored data to pass through firewalls using some of the following techniques. An important application of this security entails communication of confidential information and hidden data storage to allow necessary certification data sent from hospital to hospital. Since it provides access control system, only the owner of the document can view it as there is a unique ID on them. Further, it is very useful in copyright material, medical image processing where personal details embedded in the report, used in TV broadcasting. Notable Steganography methods utilized in a telecommunication network is the IP telephony. It hides information in any layer using the TCP/IP protocol stack. Secondly, is the spatial domain, adaptive method and frequency domain. The space area works on least significant bit (LSB) while the frequency domain merges secret messages using DCT (discrete cosine transform) and lastly, the adaptive field works a hybrid of the two methods.
Medical Imaging Segmentation Example
The most important part of medical image processing is image segmentation. According to Norouzi et al., (2013, p.199) image segmentation is a procedure for extracting the region of interest (ROI) through an automatic or semi-automatic process. Many image segmentation methods have been used in medical applications to segment tissues and body organs. Some of the applications consist of brain development study; functional mapping and blood cells automated classification.
In brain tissue segmentation different tissues are separated from each other, through extracting and classifying features such as image pixels into anatomical regions which may be useful in extracting bones, muscles, and blood vessels. Thus the segmentation of brain tissue partitions the image into different region colours such as white which can be useful in identifying the cerebrospinal fluid in brain images, white matter, and grey matter. This aids in detection and treatment of neurosurgical diseases (Balafar and Ramli et al. 2010, p.261). MRI is the applicable segmentation method MRI due to its high contrast which is made of a mixture of three techniques that is the double mathematical morphology, the active contour model and maximization segmentation. These methods are custom made for specific brain tissue such that every result is fit. Additionally, through MRI, different types of neuropathology and different tissues are pictured in different levels. It is this recognition that is applied in mapping of practical activation into brain anatomy, used in assessment of neuroanatomical inconsistency in normal brains and not to mention its use in the study of brain development. (Gordillo et. al, 2013, p. 1428).
Bibliography
Ald- Mour, H, Al-ni. A (2016) Quality optimized medical image information hiding algorithm that employs edge detection and data coding: Journal of Electrical, Mechanical and Mechatronic Systems, Faculty of Engineering and Information Technology, University of Technology Sydney: Australia 24–43
Balafar.M.A et al (2010) Review of brain MRI image segmentation methods: Published online: 22 January 2010; pp 261–274
Deserno. M.T et al (2013) Viewpoints on Medical Image Processing: From Science to Application; Current Medical Imaging Reviews, pp. 9, 79-88 79
Deserno. T.M. (2011) Biomedical Image Processing, Biological and Medical Physics,
Gordillo. N et al (2013) State of the art survey on MRI brain tumor segmentation: Journal of Magnetic Resonance Imaging 31; pp. 1426–1438 .www.mrijournal .com
Mohapatra, C, Pandey, M (2015A) Review on current Methods and application of Digital image Steganography: International Journal of Multidisciplinary Approach and Studies. Volume 02, No.2.
Nandi, D et al (2015) Principal component analysis in medical image processing: a study: Vol. 1, No. 1, (65-86)
Rahan. K, Kumar, A, Bhargava (2014) Review: Existing Image Segmentation Techniques; International Journal of Advanced Research in Computer Science and Software Engineering: Volume 4, Issue 4, pp 153-156: Available online at: www.ijarcsse.com.
Cite this page
Essay Sample on Medical Imaging Segmentation. (2018, Jan 24). Retrieved from https://speedypaper.net/essays/medical-imaging-segmentation
Request Removal
If you are the original author of this essay and no longer wish to have it published on the SpeedyPaper website, please click below to request its removal:
- A Cultural Theme in The Spirit Catches You, And You Fall Book
- Capital Budgeting Case Study, Free Paper Example
- Biology Essay Example: Respiration and Photosynthesis Cycle
- Essay Sample on Russian 19th Century Literature
- Essay Sample with the Focused Assessment: Behavioral and Mental Health
- Free Essay: Application of My Personal Model (Servant Leadership Model) in Nursing Leadership
- Free Essay on Racial Issues in Urban and Suburban Areas
Popular categories