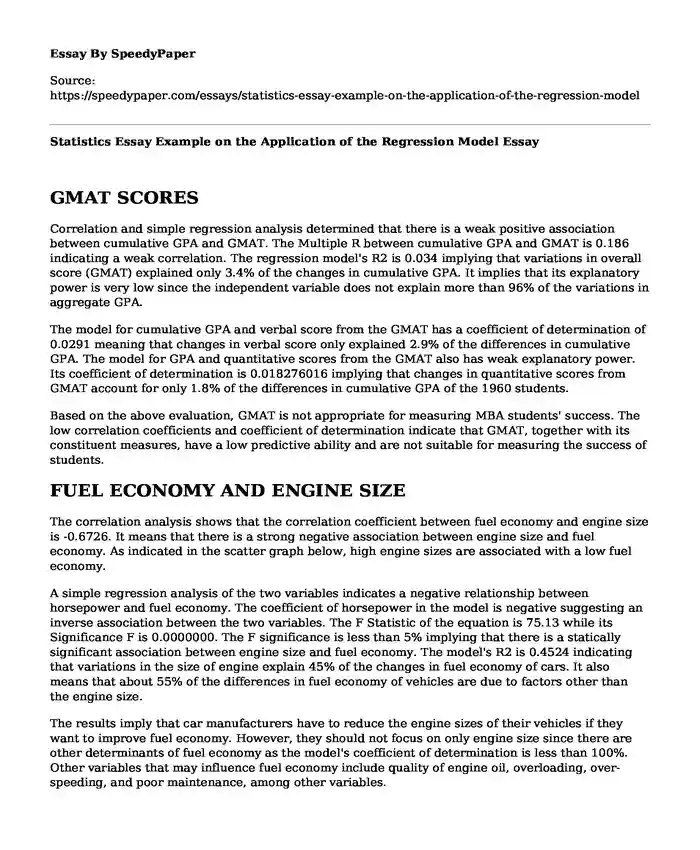
Type of paper:Â | Course work |
Categories:Â | Statistics |
Pages: | 4 |
Wordcount: | 1004 words |
GMAT SCORES
Correlation and simple regression analysis determined that there is a weak positive association between cumulative GPA and GMAT. The Multiple R between cumulative GPA and GMAT is 0.186 indicating a weak correlation. The regression model's R2 is 0.034 implying that variations in overall score (GMAT) explained only 3.4% of the changes in cumulative GPA. It implies that its explanatory power is very low since the independent variable does not explain more than 96% of the variations in aggregate GPA.
The model for cumulative GPA and verbal score from the GMAT has a coefficient of determination of 0.0291 meaning that changes in verbal score only explained 2.9% of the differences in cumulative GPA. The model for GPA and quantitative scores from the GMAT also has weak explanatory power. Its coefficient of determination is 0.018276016 implying that changes in quantitative scores from GMAT account for only 1.8% of the differences in cumulative GPA of the 1960 students.
Based on the above evaluation, GMAT is not appropriate for measuring MBA students' success. The low correlation coefficients and coefficient of determination indicate that GMAT, together with its constituent measures, have a low predictive ability and are not suitable for measuring the success of students.
FUEL ECONOMY AND ENGINE SIZE
The correlation analysis shows that the correlation coefficient between fuel economy and engine size is -0.6726. It means that there is a strong negative association between engine size and fuel economy. As indicated in the scatter graph below, high engine sizes are associated with a low fuel economy.
A simple regression analysis of the two variables indicates a negative relationship between horsepower and fuel economy. The coefficient of horsepower in the model is negative suggesting an inverse association between the two variables. The F Statistic of the equation is 75.13 while its Significance F is 0.0000000. The F significance is less than 5% implying that there is a statically significant association between engine size and fuel economy. The model's R2 is 0.4524 indicating that variations in the size of engine explain 45% of the changes in fuel economy of cars. It also means that about 55% of the differences in fuel economy of vehicles are due to factors other than the engine size.
The results imply that car manufacturers have to reduce the engine sizes of their vehicles if they want to improve fuel economy. However, they should not focus on only engine size since there are other determinants of fuel economy as the model's coefficient of determination is less than 100%. Other variables that may influence fuel economy include quality of engine oil, overloading, over-speeding, and poor maintenance, among other variables.
SPENDING ON EDUCATION AND NUMBER OF PRISONERS
Relationship Between Education Expenditure and the Number of Prisoners
The regression model for education expenditures and prisoners' population shows a positive relationship between the two variables. This is because the coefficient of prisoners in the model is 0.23. The scatter plot below also suggests a positive association between state-level education spending and number of prisoners. Its Multiple R is 0.925134914 indicating a strong positive correlation between the variables.
The coefficient of determination of the model, as shown by R2, is 0.85587. It means that changes in prisoners' population explain 86% of the variations in state-level education spending. This implies that the regression's explanatory power is high since only 14% of the variations in education spending are due to variations other than prisoners' population. The F Significance of this regression is 0.00000 indicating that at 95% confidence level, the association between education spending and the number of prisoners is statistically significant.
Relationship Between Per Capita Education Expenditure and the Per Capita Number of Prisoners
The coefficient of prisoners per capita is -0.001010186 indicating an inverse association between per capita education spending and prisoners per capita. The pattern of the scatter plot below also suggests a negative relationship between the two variables. The Multiple R is 0.006923344, implying there is almost no correlation between the two.
The R Square of this model is 0.0000479327 implying that changes in per capita prisoners' numbers accounted for 0.000% of the variations in per capita education expenditure. It implies that the model's explanatory power is very low (close to zero). The significance F for the equation is 0.961941185. This is greater than 5% indicating that the association between per capita state-level education spending and per capita prisoners' population is not statistically significant.
Most Appropriate Regression
The most appropriate regression is that between education spending and prisoners. This is because it has a greater explanatory power (coefficient of determination) than that of the regression between per capita education spending and per capita prisoners' population. Besides, it is statistically significant while that of the per capita variables is not statistically significant. Therefore, education spending depends on the actual prisoners' population and not per capita prisoners.
HOUSING MARKET STUDY
The size of a house and age are some of the factors influencing the price or value of a house. The regression model for selling price and square footage shows that a high square footage is associated with a high selling price. The multiple R for the model is 0.83 suggesting a strong positive correlation. Besides, the coefficient of square footage in the equation is positive. Its R2 is 0.6964 implying that changes in square footage explain 69.6% of the variations in selling prices of houses. The equation's F Significance is 0.0000 indicating that the association between selling price and square footage is statistically significant.
On the other hand, the regression model for the association between selling prices and age of the house shows a negative relationship between the two variables since the coefficient of age is negative. Its Multiple R is 0.66 showing a strong positive correlation between the variables. The coefficient of determination is 0.43 meaning that variations in age account for 43% of the fluctuations in hose selling prices. The F Significance is less than 5% meaning that the model is statistically significant.
The above analysis shows that both age and square footage and are significant variables influencing the price of a house. Square footage is a better fit for selling prices since its model has a higher coefficient of determination than age. However, real estate developers should consider both factors.
Cite this page
Statistics Essay Example on the Application of the Regression Model. (2022, Apr 25). Retrieved from https://speedypaper.net/essays/statistics-essay-example-on-the-application-of-the-regression-model
Request Removal
If you are the original author of this essay and no longer wish to have it published on the SpeedyPaper website, please click below to request its removal:
- Essay Example on the Elite Book Holder Project
- Free Essay on South Africa Economy
- Essay Sample on The Adventures of Robin Hood Movie
- Free Essay Example: Exposing the Truth About Guns
- Escaping Freedom - Essay Example about Independence in Marriage
- Essay Sample. Relationship Between War and Literature
- Essay Sample on Drug Addiction Treatment
Popular categories